Machine learning offers the promise to train models that perform surprisingly well on a wide range of tasks. It is an open question, however, what these models actually learn about their training data, what an adversary might be able to learn from different levels of
access to the model, and how we can effectively audit a model for privacy risks. In this talk, I will discuss disclosure risks associated with machine-trained models, with a particular focus on surprising (and potentially harmful) things a model may reveal not
just about individual training records but about the distribution of its training data. I will also delve into how well traditional
approaches to measuring privacy leakage can work for generative models such as Large Language Models (LLMs), and ideas for new strategies to audit generative AI models. I’ll conclude with some thoughts on why defending against these types of attacks is hard, why formal notions of privacy fail to capture important privacy issues, and what we might learn about how we should be training and exposing models.
Time: 16 October 2024 at 12:30 pm
Place: Science Auditurium I
Presented by Prof. David Evans of the University of Virginia.
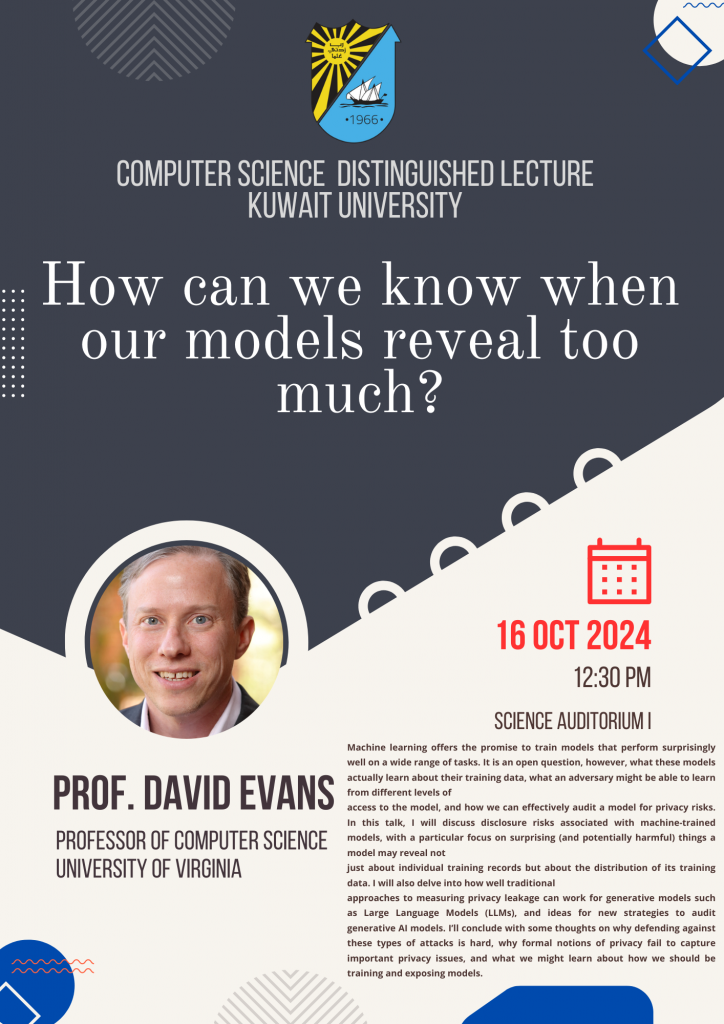